Open data has been a topic widely discussed among researchers and research-supporting organizations over the last decade. Much progress has been made in data sharing, and we now have more datasets openly available than ever before. At the same time, while in some circles the discussion about practices for data sharing and reuse may have seemed ubiquitous, the reality is that adoption still varies substantially, across research communities and across sectors.
This year’s Researcher to Reader conference provided an opportunity to take stock of progress made to date, and to explore what areas still need attention in the research data ecosystem. As part of the conference, we facilitated a workshop focused on the current barriers and potential solutions to advance research data sharing and reuse.
The workshop brought together representatives of publishers, librarians and research ecosystem vendors. Importantly, the workshop also provided dedicated time to hear insights from a couple of researchers on their perspective and practices with data sharing and reuse: Shozeb Haider (University College London) and Mauricio Contreras (The Sainsbury’s Lab). Both researchers viewed data sharing as integral to their research practice, and highlighted the crucial role of open data in increasing reproducibility and trust in research.
🚩 The data lifecycle
The workshop kicked off with a discussion around the data lifecycle and where research data can be shared and reused by different stakeholders (i.e researchers, funders, institutions, publishers, etc.) during the research process. Drawing on the perspectives shared by the researchers and the attendees’ broader experiences with research data, the discussion highlighted the importance of data management plans as a key item to support best practices in data sharing and policy compliance. Turning to data reuse, metadata and contextual information were noted as critical to enable replication and inclusion of others’ data in new research projects. The need for incentives also received attention, with calls for improvement to the connections between datasets and other outputs at all stages of the research process, in order to facilitate attribution and credit for the researchers who share their data.
🚧 Challenges in data sharing and reuse
The workshop then moved to a deeper exploration of current barriers for the adoption of data sharing and data reuse practices. The discussion brought about a diverse set of potential barriers, some related to items highlighted in the initial conversations, along with additional nuanced considerations related to peer or community expectations and legal or privacy restrictions. The different items raised clustered into three broader themes:
- Lack of credit or incentives for sharing or reusing data, and fear of scooping (i.e., the risk of another group making use of shared data, and publishing results based on it before the producers of the data do so)
- Lack of consistent community standards
- Need for greater support for researchers, in the form of training, dedicated expertise in data management, and funds to support costs associated with data management and sharing
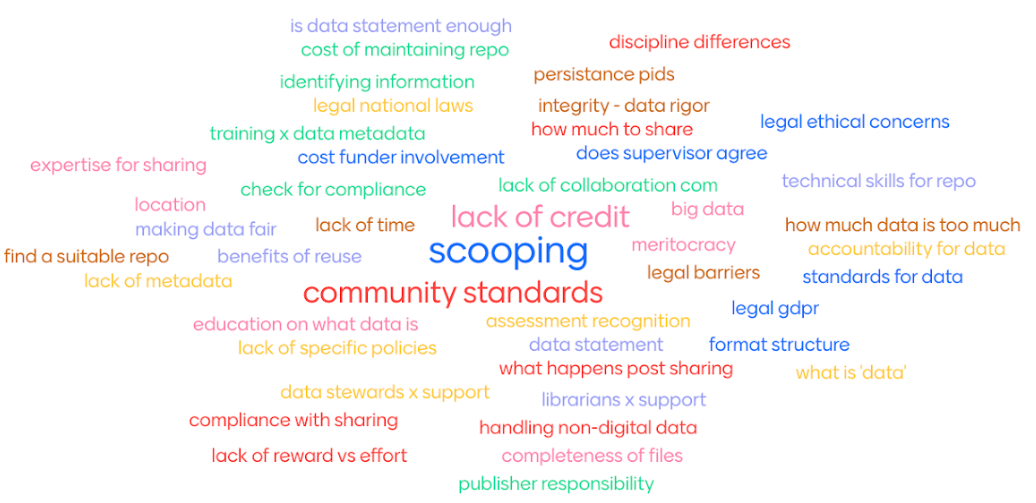
Figure 1. Word cloud highlighting barriers for data sharing and reuse identified by the workshop participants.
🛣️ The path ahead
Having an understanding of the existing challenges, we moved into a solutions-oriented discussion where we asked attendees to propose solutions to the main themes identified. As part of the exploration of the different proposed solutions, we categorized ideas according to the perceived potential impact and effort involved in driving that solution forward.
The group identified a couple of areas as having potential impact but requiring lower effort, likely reflecting their level of development in the open data ecosystem. The first area related to the infrastructure for data sharing and reuse, as well as that required for collecting data metrics and relational metadata between datasets and other outputs. Infrastructure for research data has developed substantially in recent years, with a range of repositories available to host, curate, preserve (and where necessary manage access) for research datasets. Tools and services are also available to collect information on data usage, e.g. DataCite provides services to connect datasets to other outputs and aggregate data usage metrics, and the Make Data Count initiative has developed guidance to consistently report measures of data usage. The second topic with similar categorization of the required effort focused on support for researchers, in the form of training and dedicated roles that support data management and stewardship. This is also an area that has advanced over the last decade, with several institutions (e.g. TU Delft) implementing data steward programs and data-management support for researchers.
On the other hand, a number of solutions raised during the discussion were perceived as bringing high impact but also requiring substantial effort. These involved: 1) updates to research assessment frameworks to recognize open data practices and create incentives for data sharing and data reuse, and 2) greater consistency in standards and community expectations for data sharing, while accounting for the specific needs of individual communities. Overall, the group saw value in exploring additional ways to support the community in their diverse journeys toward adoption of open data practices.
Drawing on the discussions at the workshop, we developed recommendations for how different actors in research data can drive forward data sharing and reuse, summarized in the table below. We welcome feedback on these recommendations, as well as additional examples of groups, institutions or initiatives who are already driving these areas forward.
The recommendations are multiple and varied, and we recognize that some communities may have already completed or may be actively working on some areas, while others will be at earlier stages of implementation. It is not too late to join the journey. We invite you to consider the recommendations, identify one key area that you can work on, and champion its adoption within your organization and your community. Every step counts!
You can read the full white paper summarizing the discussions at |
Recommended actions |
Examples |
FUNDERS |
|
|
NIH Data Management and Sharing Policy Horizon 2020 Data Management Guidance |
INSTITUTIONS |
|
|
TU Delft Data Stewardship programme EMBL guidelines for research assessment (which mention data) Puebla et al., Ten simple rules for recognizing data and software contributions in hiring, promotion, and tenure Coalitions for reforming research assessment: CoARA, HELIOS Open |
REPOSITORIES |
|
|
Dryad’s best practices for creating reusable data publications NIH Generalist Repository Ecosystem Initiative Dataverse documentation to enable the set up of the Make Data Count metrics in data repositories Make Data Count recommendations, including the COUNTER Code of Practice for Research Data for normalized reports of data usage |
PUBLISHERS |
|
|
Cousijn et al., A data citation roadmap for scientific publishers Updates to eLife’s data sharing policies Springer Nature’s Data Availability Statements guidance Muench A., The Roles of Data Editors in Astronomy |
SCHOLARLY SOCIETIES (may also be publishers, in which case see above) |
|
|
FASEB DataWorks! (provides data prizes, educational webinars and helpdesk support) Research Parasites awards for rigorous secondary data analysis Special Issue: Secondary analysis of large quantitative datasets at the Journal of Intellectual Disability Research |
Copyright © 2024 Iratxe Puebla. Distributed under the terms of the Creative Commons Attribution 4.0 License.