Since its release towards the end of 2022, ChatGPT has been dominating the majority of AI-related conversations on social media. One could almost say it has made AI more mainstream and accessible than ever. AI is quickly revolutionizing the modern-day research landscape. According to a CSIRO report, nearly 98% of scientific fields use AI in some way. The possibilities are endless, with these state-of-the-art AI technologies becoming more and more accessible.
AI tools are gradually making inroads into the research ecosystem. From breaking down research papers to make them more comprehensible and auto-completing academic essays to accurately predicting 3D models of protein structures, AI is streamlining multiple aspects of scholarly pursuit. In short, it can dramatically reduce the time researchers invest in routine tasks, giving them more time to think, focus on data and analysis, synthesize their thoughts, and make inferences.
This blog post is part of a series on how AI is reshaping the research landscape. In the first part, we will set the scene by examining the different ways AI applications are currently used in consuming and delivering research.
1. Knowledge discovery: Getting through papers faster
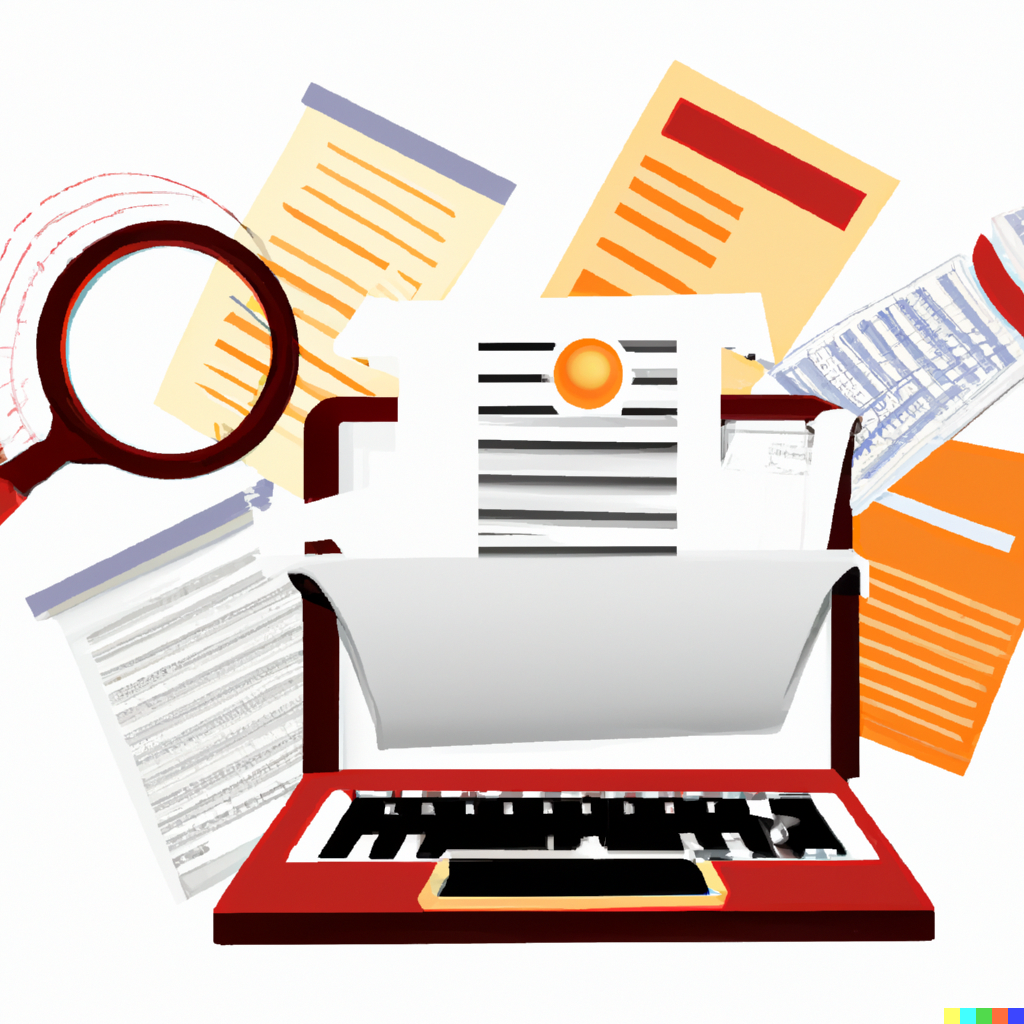
Gathering valuable insights from the sea of scientific manuscripts can be a daunting task. Given the sheer number of papers published each year — with close to 2.4 million annually — finding relevant papers and distilling critical insights from them is almost like finding a needle in a haystack.
It's a challenging feat for any researcher as there will always be unfamiliar terms, concepts, theories, and equations to cross-reference to understand the paper thoroughly. Plus, there may be questions that one would have to look up separately while making the connections between concepts. The difficulty further increases if you are a non-English speaker since three-quarters of science and humanities papers are written in English.
Fortunately, we now have AI-powered research reading tools that can help us navigate the vast array of papers and make sense of their content. SciSpace Copilot is one such tool. It helps to read and understand articles better by providing explanations for scientific texts and math, including follow-up questions for more detailed answers in multiple languages. Elicit allows researchers to access relevant papers with summarized takeaways. System is an open data resource that combines peer-reviewed articles, datasets, and models to help you understand the relationship between any two things in the world.
The list is growing, with more coming up every day. These tools aim to help researchers and science practitioners extract critical information and required context from research papers faster.
2. Communication enhancement: Articulating yourself better
Writing grant applications can take up a substantial amount of time, even for the most accomplished researchers. Some report that up to 50% of their time is dedicated to this process. On top of this, you have papers, emails, conference presentations, and even social media posts to write to disseminate your findings and make your research visible. While this is an important activity for advancing research, it is eating into the time you would spend refining your research and honing your analysis.
Generative AI model-based writing tools are tackling this challenge. A researcher used GPT-3, a large language model, to write an academic paper — complete with references. While it is probably not a good idea to use AI to write the whole piece, one can use it to bootstrap, explore different angles, and improve the content's tone, structure, and flow.
Lex is a word processor like Google Docs but interactive and AI-powered. Writefull X is an AI-powered writing application tailored to academia. Both help lighten the load, allowing you to focus on sharing your research findings rather than stressing about the actual writing.
3. Data analysis acceleration: Making sense of data faster
You can only analyze data when it is cleaned and organized. It means spending hours manually sorting and categorizing your data, which can be tedious, especially when dealing with large volumes of unprocessed data. On top of that, you might have to learn to use spreadsheet software and databases and, in some cases, coding languages like Python or R.
Thankfully, advancements in AI have made it possible to make sense of data faster and with less human effort. A wide range of AI tools that are currently available could help you each step of the way, from data extraction to data visualization and even predictive analysis.
Starting with AI-based spreadsheet bots that turn your instructions in natural language into a spreadsheet formula. So, suppose you want to find out the total number of survey respondents who answered 'yes' to a question in the age bracket 16-25. In that case, you could type the same (with column numbers), and the spreadsheet bot will create the formula to give you the answer you need. If you want to visualize the data, you have platforms like Olli that help you create line charts, bar graphs, and scatter plots by simply describing what you want.
It doesn't end there. OpenAI Codex is an AI model that translates natural language into code. This has been used to build GitHub's AI coding assistant, which gives you code suggestions in real-time, right from your editor. An MIT-based study revealed that you could use this model to solve university-level math problems consistently.
There are also AI-driven data analysis tools out there, like Lookup. You can upload the data, ask questions in plain English, and get answers quickly without learning complicated query languages or figuring out how various tables connect.
4. Publishing efficiency: Expediting the workflow
Getting a scholarly manuscript published is, again, a tedious process, with formatting, editing, proofreading, and the all-important peer-review cycle. On the one hand, you have authors spending 52 hours a year on average on formatting. On the other, journals reject around 75% of manuscript submissions before they even reach the peer review stage. These numbers indicate that there is room for improvement in the publishing workflow.
Integrating AI tools by both authors and publishers can streamline this process. On the author's side, AI-based solutions like Grammarly, Lex, Turnitin, and Writefull automate formatting, referencing, plagiarism checking, and grammar checks.
Journal publishers are also turning to AI to streamline the review process. For instance, American Association for Cancer Research (AACR) uses Proofig to verify the authenticity of images in submissions sent to their journals. Springer Nature adopted UNSILO, an AI-based platform, to identify links across eleven million published journal articles, enabling them to find related articles quickly. Penelope.ai is another AI-based tool that helps ensure that manuscripts meet a journal's requirements by quickly analyzing references and structure. AI is also being used for fact-checking. The potential for AI to optimize the journal publishing process is immense.
Final thoughts
AI models hold tremendous potential for the scientific research community. At the same time, there are serious concerns about employing such technology, ranging from plagiarism and ethical issues to the potential for replicating human biases, spreading false information, and ethics violations. Research teams and other stakeholders must join forces to guarantee that Artificial Intelligence-driven research systems are responsibly constructed and used.
AI is still evolving, and expecting it to always produce reliable results is unrealistic. After all, it's only been around five years since the release of Attention is all you need, a groundbreaking paper that introduced Transformer — an NLP model considered the foundation of many of today's AI models. Fortunately, the early signs of progress are encouraging, and continued developments are anticipated. We could expect better generation capability and factual consistency from the Large Language Models in the near future.
Even so, AI can still create inaccurate output. So, when employing AI in your workflow, ensure to double-check all outcomes before relying on them.
In the next edition of this series, we will look at how AI is helping researchers overcome language barriers. Stay tuned! Thanks for your time in reading this post. Please feel free to contact us at saikiran@scispace.com, for any questions or thoughts. All the images in this post are created with Text2Image AI tool DALL·E 2
Copyright © 2023 Saikiran Chandha, Sucheth R, Tirthankar Ghosal. Distributed under the terms of the Creative Commons Attribution 4.0 License.